Recent Research on the Harmonization of Neuroimaging Data Using RAVEL and ComBat
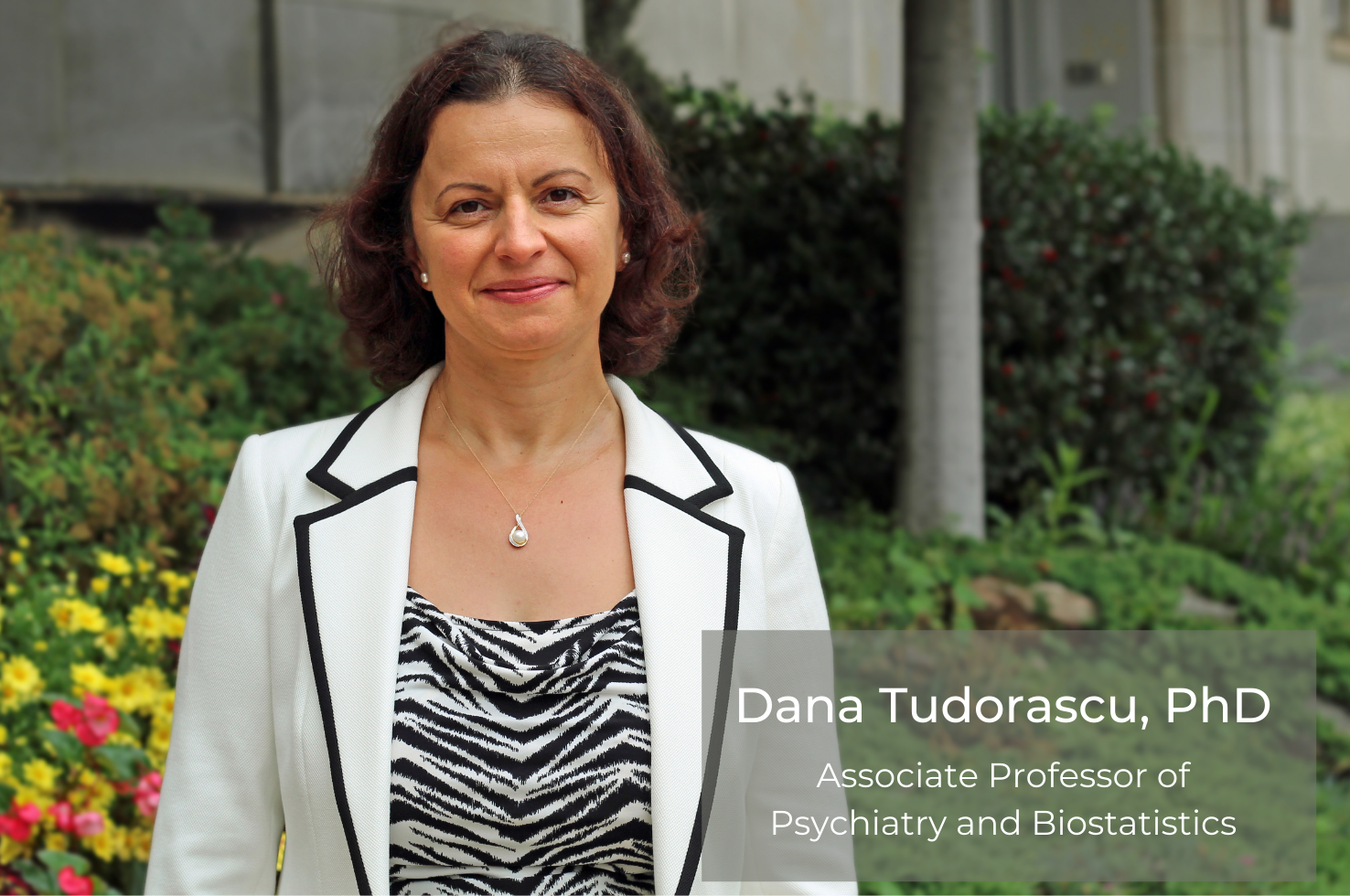
Neuroimaging studies of structural magnetic resonance imaging (MRI) provide invaluable insights into the changes underlying neurodegenerative diseases, such as Alzheimer's disease, but frequently combine data collected from multiple scanners and experimental conditions. These conditions can yield data containing substantial technical variability and biases due to differences in sites, scanners, and image acquisition protocols. In a recent study published in NeuroImage, Mahbaneh Eshaghzadeh Torbati (PhD student, University of Pittsburgh School of Computing and Information) and Pitt Psychiatry scientists Ann Cohen, PhD (Associate Professor of Psychiatry); Howard Aizenstein, MD, PhD (Charles F. Reynolds III and Ellen G. Detlefsen Endowed Chair in Geriatric Psychiatry and Professor of Bioengineering and Clinical and Translational Science); William Klunk, MD, PhD (Distinguished Professor of Psychiatry and Neurology and Levidow-Pittsburgh Foundation Chair in Alzheimer's Disease and Dementia Disorders); and senior author Dana Tudorascu, PhD (Associate Professor of Psychiatry and Biostatistics), evaluated and compared results of data analysis methods without any data transformation (RAW), with intensity normalization using RAVEL, with regional harmonization methods using ComBat, and a combination of RAVEL and ComBat.
“The main goal of our study was to eliminate variability due to scanner differences. The problem with MRI data is that you can scan the brain of the same individual on two different scanners and get very different images, because MRI measurement units are arbitrary. RAVEL is a method of standardization (normalization) that can remove inter-subject variability at the level of brain images. ComBat, on the other hand, is a harmonization method that is applied to summary measures extracted from the brain and it works by reducing variability due to the scanner,” said Dr. Tudorascu.
Methods were evaluated on sample of 16 study participants who were scanned on both 1.5T and 3T scanners a few months apart. Neuroradiological evaluation was conducted for seven different regions of interest that are pertinent to Alzheimer's disease.
Findings from the study showed that RAVEL substantially improved the reproducibility of image intensities. However, ComBat provided more consistent harmonization across subjects and image-derived measures. Additionally, RAVEL and ComBat substantially reduced bias compared to the analysis of RAW images, but RAVEL also resulted in larger variance.
“We believe that not controlling for scanner effects in multi-scanner/multi-site studies may have major implications in clinical studies. For example, if you want to measure treatment effect and you are scanning people on different scanners, you may end up with biased results. We found, for example, statistically significant cross-scanner differences for 55% of the Alzheimer’s disease-related brain regions that we studied. After applying RAVEL and Combat, the number of these regions with scanner bias got reduced by 54%,” said Dr. Tudorascu.
A multi-scanner neuroimaging data harmonization using RAVEL and ComBat
Eshaghzadeh Torbati M, Minhas DS, Ahmad G, O’Connor EE, Muschelli J, Laymon CM, Yang Z, Cohen AD, Aizenstein HJ, Klunk WE, Christian BT, Hwang SJ, Crainiceanu CM, Tudorascu DL
NeuroImage (2021). https://doi.org/10.1016/j.neuroimage.2021.118703